A Data-First Blueprint to a Helpful AI Assistant
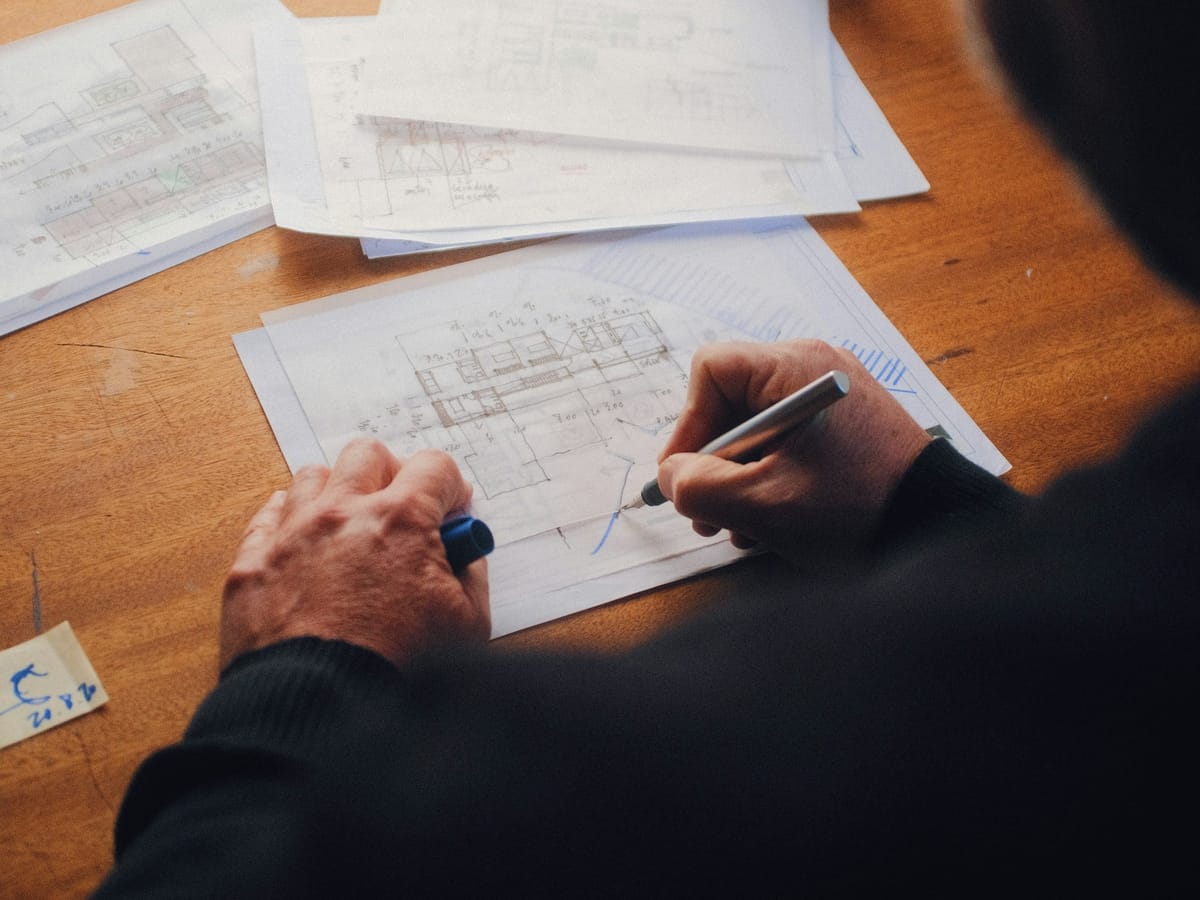
When Dawn Armstrong at HumanGood first shared her team's struggles with their internal AI assistant, it wasn't the code or the AI itself that was the problem. The culprit? Data—not a lack of good data—but an overabundance of irrelevant or outdated data.
This revelation sparked a crucial decision in our collaboration: start small, with a subset of clean data, and expand intentionally. Now, with HumanGood deciding to partner with Mellonhead to develop an AI assistant for their 5,000+ employees, this data-centric strategy is unveiling precisely what employees need for the AI to be truly useful.
Consider these tips before you rush to implement an AI assistant with your internal wiki or SharePoint instance.
1. Identify Your Data Needs
Start by diving deep into your organization's information ecosystem:
- Survey your people: Ask employees what they'd want from an AI assistant. What questions do they struggle with daily? What information is hard to find? This isn't just a wish list – it will give you a blueprint you can use for your data strategy, testing, and employee training.
- Engage stakeholders: Work with team leads across departments. What slows their teams down? Do they field repetitive questions from other employees? These pain points are goldmines for AI assistance opportunities. Find out what resources are well documented and where they have gaps. Identify the systems their team uses to answer questions.
- Prioritize and plan: Based on your findings, create a prioritized list of data needs. Focus on high-impact, frequently accessed information first. This will ensure that your AI assistant delivers value from day one.
2. Gather Your Data Intentionally
- Curate your documentation: Rally each department to gather those how-to guides, SOPs, and FAQs. They know which of their documents are most valuable and up-to-date and can help you avoid pulling in irrelevant and outdated documents.
- Turn conversations into content: Record subject-matter-experts onboarding a newbie, then transform the transcript into structured documentation. Extra credit if you have a template or existing documentation to use as a sample for formatting and level of detail. Here's an example prompt where X is a topic like "new client onboarding" and Y is a type of document like "How-to Guide", "FAQ" or "Standard Operating Procedure (SOP)".
Act as a technical writer. Extract the details from this meeting on X topic and convert into a Y. ignore details that are not relevant to the topic.”
- Create a learning loop: Set up a Slackbot to capture Q&A interactions.
3. Connect with Caution
While it's tempting to plug your AI into every system, slow and steady wins the race. Focus on answering static questions well and pointing employees to external systems for more complex or personalized answers.
Once you have good coverage and quality responses, then begin integrating other sources that will be impactful for employees:
- APIs can provide a safe gateway to internal and external systems until we live in a world where your Internal Company AI assistant can ask a vendor’s agent a question.
- CSV and Excel: LLMs made huge strides in 2023 and 2024 to gain insights and extract information from structured data. However, indexing and analyzing an unbounded number of these documents adds complexity—save this for a second or third phase.
- Prioritize security: Systems with PII, financial, or health data, like invoicing or benefits, should be last to integrate and require security, privacy, and compliance by design.
Embrace the Evolution
Your AI assistant should be a living, learning entity. Set up a way for users to flag outdated or incorrect info. This crowd-sourced quality control will be the key to keeping your assistant helpful as time passes.
Success with an internal AI assistant isn't about drowning it in data. It's about feeding it the correct information, bit by bit. Start small, stay focused, and watch your AI become an indispensable tool for your team.