Cracking the AI Black Box: Explainable AI
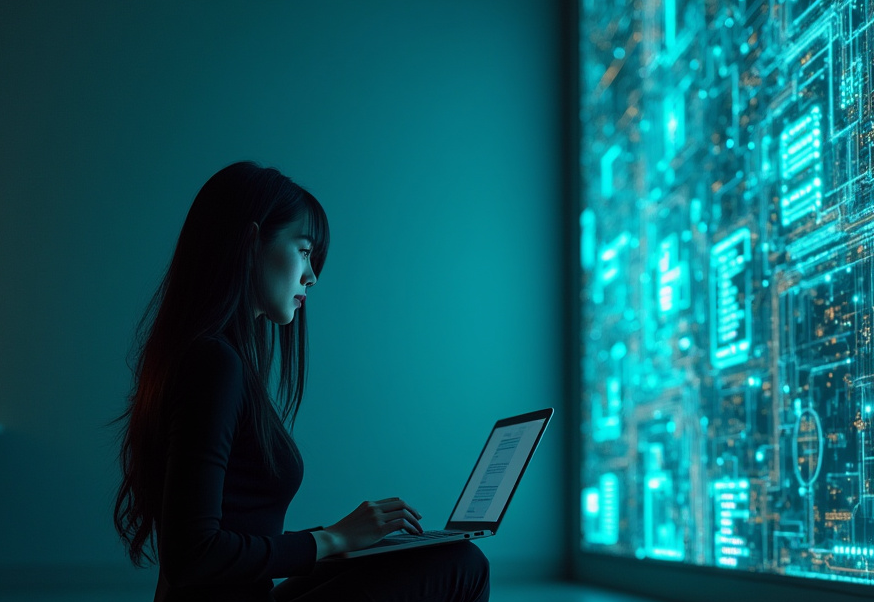
Hello again, AI enthusiasts!
What if I told you your AI assistant has a 'thought process' we're just beginning to understand? I spent years trying to determine how feature weights influence the output of ML models so that I could “explain AI” to customers. There wasn’t and still isn’t an easy way to understand AI responses, but there is a lot of progress in this area of research.
I’ll share some of these breakthroughs and practical tips for understanding AI decisions in today's newsletter. But first, some exciting news.
I’ve just opened registration for my final course of the year, "Getting More From Generative AI." It's designed for those frustrated with ChatGPT's hit-or-miss results. In four sessions, you'll learn new AI tools and prompting techniques for to improve how you brainstorm, research, write, and analyze data. The course runs in late October, and videos are available for those who can’t make live course sessions. Ready to enroll? Register online. Use promo code LEVELUP before end of month for a discount.
Now, let's unpack the mystery of AI decision-making...
Breakthroughs in The Black Box
For years, the inner workings of neural networks have been notoriously difficult to interpret. Even understanding a single layer has been a significant challenge for researchers and practitioners alike. However, recent advancements are starting to shed light on these complex systems.
The announcement of Google's Gemma Scope is what piqued my interest. Using 400 sparse autoencoders (SAEs), it interprets every layer of the LLMs powering Gemini. Think of SAEs as AI translators, decoding the neural network's "thought process."
Previous research had used a small number of SAEs, but 400 — that’s a big leap forward.
These advancements could lead to more reliable and advanced AI technologies. But while researchers work on cracking the black box, what can you do now?
Practical Approaches to Understanding LLM Outputs
Here are a few quick tips for understanding LLM outputs
- Spot Patterns: LLMs mirror input patterns. Unexpected output? Consider what patterns the model might be seeing.
- Think in Probabilities: LLMs don't have single "right" answers. They sample from probability distributions, explaining varied responses to the same question.
- Craft Smart Prompts: Your question framing influences the output.
- Know Your Tools: APIs and AI products often pre- and post-process LLM responses. Understanding these modifications can explain unexpected results.
We can gain valuable insights into LLM outputs by employing these approaches, even without full explainability.
The Road Ahead
As AI explainability (XAI) evolves, we're better positioned to design responsible systems, implement effective guardrails, and continuously improve AI performance. We can develop and implement AI systems today with what we know about how to influence LLM responses:
Understanding the inner workings of AI models allows us to make more informed decisions about system architecture. For instance, we can better choose between using retrieval-augmented generation (RAG) systems or fine-tuning LLMs based on our specific needs and explainability requirements.
While we can't fully decode an LLM's "thoughts," these tools help us make sense of their outputs.
Schedule a consultation to see how Mellonhead can help you execute your AI strategy.
or, if you want to level up your skills with Generative AI tools sign up for my upcoming course, "Getting More From Generative AI." Use promo code LEVELUP before end of month to get a discount.